In WASP we are curious and experimenting with different types of scientific collaborations. We firmly believe that openness is a key in creating scientific excellence.
The research within WASP is conducted towards several different goals. Many of our research projects aim to build broad ground theory, some are narrow and breaching the very frontier of our knowledge, others are somewhere in between. Therefore, we are conducting devoted research projects with different types of collaborations. On this page you can read more about them.
NEST Environments
The NEST initiative is a major investment in multidisciplinary research environments and networks. The underpinning idea is to address hard, challenging research questions that require multidisciplinary efforts. Nine projects were awarded grants in the first NEST-call, 2021.
WASP-DDLS Collaboration Projects 2022
During 2022, WASP and the SciLifeLab and Wallenberg National Program on Data-Driven Life Science (DDLS), the two largest research programs in Sweden, launched a second joint call with the aim of solving ground-breaking research questions across their different scientific disciplines. In total, 13 applications were awarded grants for two-year projects.
Researchers
Nina Linder (UU), Claes Lundström (LiU)
Background
Cervical cancer is the fourth most common cancer among women worldwide, and about 90% of the new cases and deaths occur in low- and middle-income countries. Linder et al have previously developed an AI-based point-of-care (POC) diagnostic system for cervical cancer screening in resource-limited settings. However, domain shift poses challenges for large-scale implementation of AI-based diagnostic methods, as AI systems may yield unreliable predictions when encountering data that differs from the training data.
Aim
To develop computational methods based on uncertainty estimation, to tackle domain shift challenges in AI applications for digital cytology and to integrate the methods into a workflow in a prospective study for improved human-and-machine interplay in the diagnostic process.
Methods
The project will explore methods to mitigate the domain shift challenges, focusing on uncertainty estimation to increase prediction robustness or detect mispredictions. A prototype clinical viewer, including uncertainty estimations will be evaluated within a prospective clinical study. The assumption is that uncertainty predictions will allow human experts to focus on challenging cases, while high-confidence AI predictions require minimal human intervention.
Significance
The project has significant societal and industrial impact potential by enabling more accurate, efficient, and accessible diagnostics for cervical cancer, also in resource-limited settings.
Researchers
Volker Lauschke (KI), Ming Xiao (KTH)
Background
Chemotherapy is essential in the treatment of several cancers; however, drug resistance remains a substantial problem. To predict chemotherapy response, certain genetic variations in the drug transporter genes MDR1, MRP1 and BCRP are currently used as clinically established biomarkers. In a recent study, genomic data from >130,000 individuals were analyzed, revealing more than 3000 novel genetic variations in these transporters with unclear functional consequences.
Aim
Develop a machine learning based tool for pharmacogenetic predictions of chemotherapy response in cancer treatment.
Methods
A substrate-specific functionality profile of all genetic variants of human drug transporters will be generated using deep mutational scanning followed by phenotypic selection in cancer cells. The resulting data set will be used for the development of graph neural network (GNN)-based learning schemes for pharmacogenetic predictions of drug response.
Significance
This project will facilitate the translation of an individual’s genomic information into chemotherapeutic sensitivity profiles, providing an important mechanistic link between rare genetic variations, transporter function and clinical outcomes. The long-term goal is to allow for more individualized therapy strategies to increase patient survival, but also to decrease the risk of severe side-effects treatments.
Researchers
Pelin Sahlén (KTH), Wojciech Chacholski (KTH)
Background
A deeper knowledge of human genetic variation and its complex interplay within genomic elements constitutes a cornerstone in the understanding of several human diseases. The 3D arrangement of the human genome reveals functional information by physically positioning regions that work in close proximity. Novel methods that can look at the 3D arrangement and covariation profiles of multiple locations in genome are highly needed.
Aim
Develop a method to improve the understanding of sequence covariation and its connection to pathology.
Methods
Sequence and functional datasets will be used to establish criteria for identifying when genetic elements, like genes, lincRNAs, promoters, or enhancers, either tend to appear together or exhibit differences in their patterns of variation. Topological Data Analysis (TDA) will be used to explore and the relative geometry of genomic topologies to find places that function together.
Significance
The long term goal is to go beyond linear analyses of the genome data by exploiting the genome folding constraints to obtain a glimpse of coordinated regulation of the genome and its impact in disease onset and progress.
Researchers
Fredrik Levander (LU), Lukas Käll (KTH)
Background
Molecular biomarkers are a prerequisite in contemporary precision medicine. Proteins are the functional entities in cellular processes; therefore, proteomics provides excellent possibilities to discover novel biomarkers. Proteoforms are different molecular forms or variants of a protein that can arise due to various post-translational modifications, alternative splicing of mRNA, or other molecular events, each of which may have distinct biological functions or properties. So far, proteoforms are to a large degree ignored in current proteomic studies, mostly due to shortcomings of current informatics workflows that fail to handle the complexity of data.
Aim
Enable the differential quantification of proteoforms in sample cohorts, by leveraging the millions of mass spectra deposited in repositories using novel feature extraction and machine learning approaches.
Methods
Spectral clustering, feature extraction and deep learning will be used to develop methods to detect modified proteins in deposited data. A machine learning framework to detect proteoform level differences within sample will be developed. The resulting algorithms will be used to reanalyze medically relevant datasets to detect novel biomarkers.
Significance
The project aims to transition proteomics from a gene-centric to a proteoform-centric perspective, thereby amplifying the opportunities to discover pertinent biomarkers.
Researchers
Björn Önfelt (KTH), Mårten Björkman (KTH)
Background
Natural killer (NK) cells are a vital part of the innate immune system through their capacity to detect and eliminate virus infected or transformed cells without prior activation. Cellular immunotherapy utilizes the immune system’s ability to recognize and kill malignant cells. For effective therapy it is important to harvest and expand immune cells that show effective anti-tumor responses. Current methods are mainly relying on isolating cells based on protein phenotype, often leading to cell populations that are very heterogeneous in terms of functional responses.
Aim
Develop new, improved methods to automate the process of identifying NK cells with extraordinary ability to kill tumor cells.
Methods
Machine learning (ML) algorithms will be trained to distinguish NK cells displaying different levels of cytotoxicity using image data from single cell screens. By increasing content in the imaging data used for training, and include time-lapse sequences revealing cell dynamics, NK cell identification will be improved. A framework for fully automated AI-driven identification and harvest of NK cells with extraordinary cytotoxic potential will be forged.
Significance
Development of a framework for fully automated AI-driven identification and harvest of NK cells with extraordinary cytotoxic potential is assumed to significantly improve the efficiency of adoptive cell therapy in cancer treatment.
Researchers
Ville Kaila (SU), Simon Olsson (Chalmers)
Background
Mitochondria are membrane-bound cell organelles generating energy supplies in the form of ATP through oxidative phosphorylation. Complex I is the largest component of the mitochondrial oxidative phosphorylation system, and its dysfunction is linked to nearly half of all known mitochondrial disorders, with point mutation resulting in e.g. cancer and neurodegenerative diseases. Despite the significant structural and biochemical data over the last decades, their mechanistic principles remain poorly understood and a major challenge for life-sciences.
Aim
Develop methods to quantitatively predict the biological reactivity and functional dynamics of the membrane proteins responsible for energy transduction in mitochondria, with focus on complex I.
Methods
Physics-based ML models will be derived using neural networks by combining highly accurate, but computationally challenging quantum simulations with biophysical experiments, evolutionary and mutagenesis data. The models aim to predict how the protein structure determines the biochemical activity and apply these to large-scale in silico-screening for probing how mitochondrial disease-related mutations alter the protein function.
Significance
The project will combine ML approaches with biochemical, biophysical, and structural data, providing a basis for understanding how proteins power the energy metabolism of our cells, the evolution that led to the emergence of these intricate biological complexes, and how human disease related mutations alter the protein function.
Researchers
Anna Rising (SLU), Hedvig Kjellström (KTH)
Background
Due to its impressive strength, material properties, and sustainability, artificial spider silk is a highly desirable material that could potentially be used in a vast number of applications. However, artificial replication of spider silk has turned out to be much harder than previously thought and artificial fibers are inferior to native silk. Recently, a new study revealed the transcriptomes from more than 1000 silk glands and corresponding data on silk fiber mechanics that might help solve this mystery.
Aim
To use machine learning on the available data to reveal the unique factors that give native spider silk its strength, and to test the results with previously developed in-house methods.
Methods
By utilizing machine learning on the data from the new study, such as expression levels, protein molecular weight, hydrophobicity, secondary structure (predicted by AlphaFold2), and amino acid residue composition, the project PIs plan to reveal the unique factors that give native spider silk its mechanical properties. The researchers will then use their own unique biomimetic method for production of artificial spider silk fibers to verify the output from the artificial intelligence, and to spin fibers with mechanical properties that match those of native fibers.
Significance
If the project is successful, the results could have a major impact on the material science field and play an important role in the sustainability transition by providing materials with low negative impact on the environment. They could also facilitate the development of other proteinaceous high-performance materials.
Researchers
Malin Malmsjö (LU), Victor Olariu Ahnell (LU)
Background
Conventional diagnosis of skin cancer involves biopsy excisions followed by histopathological analysis. This invasive process, which can be painful for patients and often results in the removal of a larger area of skin than necessary, requires trained specialists and can take several days or even weeks to complete. Hyperspectral imaging, a relatively new technique that enables the analysis of a much wider spectrum of light than what our eyes can see, and photoacoustic imaging, a non-invasive technique that converts ultrasonic wave patterns generated by laser-heated tissue into detailed spatial images, might be the key to faster, more accurate, and non-invasive diagnoses.
Aim
To develop a machine learning model that can identify tumor borders orders of magnitude faster than the current state of the art methods, by analyzing hyperspectral and photoacoustic images.
Methods
Hyperspectral imaging will be used in combination with photoacoustic imaging to be able to analyze both the spectral molecular fingerprint and depth of skin lesions. Artificial intelligence will then be trained to accurately predict the correct diagnosis.
Significance
By using neural network models on high contrast images containing unique molecular information from hyperspectral and photoacoustic images, it is possible to streamline skin tumor diagnosis, making it both safer and faster for the patients. This will also reduce the medical costs as well as lower patient suffering by reducing the number of misdiagnosis and unnecessary re-surgeries.
Researchers
Henrik Hult (KTH), Peder Olofsson (KI)
Background
Bioelectronic medicine, an emerging discipline combining neuroscience, immunology, and electrical engineering, might lead to the development of new methods capable of monitoring and treating diseases by electrical intervention in the peripheral nervous system.
Aim
To create data-driven statistical and machine learning algorithms that can analyze electrical signals from the peripheral nervous system to predict the level of glucose in the blood, and to provide proof-of-principle that an autonomous machine can replace the sensory detection by the central nervous system of bodily functions, with potential applications that will range far beyond predicting glucose levels.
Methods
The data will be collected through an implanted electrode on the vagus nerve while blood glucose levels will be varied. Using a conventional device to measure blood glucose as a reference, training data from the electrode is generated as high-frequency multi-channel signals that can be analyzed using a combination of statistical signal processing techniques and machine learning algorithms.
Significance
By utilizing artificial intelligence and machine learning to interpret the recorded nerve signals from the peripheral nervous system, autonomous adaptation of treatment could potentially be achieved. This will help tackle the well-known dosage and timing problems, which are responsible for many unwanted side effects of currently available pharmaceutical drugs. This will be an important step towards truly personalized medicine.
Researchers
Jonas Frisén (KI), Jens Lagergren (KTH)
Background
To understand human development and pathological processes, one needs to understand how different cell types are generated and how they function. It is also vital to understand what drives these cell types to develop into specific phenotypes, whether it is determined by their lineage or by their surrounding microenvironment. LUSTRE, a technique developed by the Frisén lab, can track a specific marker segment (LINE-1) inside cells to reveal information about their lineage, which could help in the development of new pharmaceuticals targeting specific cells.
Aim
To track genomic alterations and reconstruct linage trees in human blood, brain, and cancer cells, by developing and optimizing phylogenetic methodologies that take advantage of modern deep-networks to sort out the underlying signal, from LUSTRE data, and to develop Variational Auto-Encoders (VAEs) to reduce experimental noise.
Methods
LUSTRE is used to track the LINE-1 elements in single cells, while gene expression is simultaneously analyzed with Smart-seq3, to explore lineage relationships in human tissue. Deep learning neural network-based methods are then applied to track genomic alterations at the single-cell level. Mitochondrial mutational profiles will also be used in combination with LUSTRE data to identify cell families and determine spatial localization.
Significance
The study holds significant implications for understanding human development and disease processes, and might reveal important information about different cell types, which can help develop new therapies targeting these specific cell types.
Researchers
Niklas Mattsson-Carlgren (LU), Kalle Åström (LU)
Background
Neurodegenerative diseases like Alzheimer’s and Parkinson’s are serious global health problems with no effective cures. Studying these diseases directly in patients is crucial, but the disease progression is slow, and the symptoms may vary from patient to patient. Developing biomarkers is one way to detect diseases early but due to legal and ethical concerns, sharing data is challenging. To address this problem, scientists are now exploring “synthetic cohorts”, which consists of virtual patient data sets. These virtual clinical-like data sets could be of vital importance to researchers with novel methodology but lacking patient data, help train AI models, enable “in silico” experiments, and for educational purposes.
Aim
To utilize machine learning models to create realistic “synthetic cohorts” of patients with neurodegenerative diseases, which will be shareable without violating current ethical and legal principles. The data from these models will also be analyzed to reveal new associations within neurodegenerative diseases, advancing our understanding of these conditions.
Methods
The generation of synthetic cohorts involves mathematical modeling using existing observational data. These models enable the creation of comprehensive, virtual patient datasets, allowing researchers worldwide to conduct experiments related to neurodegenerative diseases.
Significance
The development and global availability of “synthetic cohorts” play a pivotal role in advancing our understanding of neurodegenerative disease mechanisms. By identifying new associations and potential drug targets, these cohorts could accelerate therapy development. The knowledge gained from the project might also have a big impact on various other areas of medicine.
Researchers
Aleksej Zelezniak (Chalmers), Enric Llorens (KI)
Background
While gene and cell therapies show great potential in treating genetic disorders by targeting inherent genetic defects, effective regulation of therapeutic gene expression in a cell type-specific manner remains a challenge. Achieving precise control over the gene expression has the potential to significantly enhance both the safety and efficacy of those therapies, reducing side effects and health risks. One way to achieve this is to utilize advanced sequencing techniques and machine learning, particularly deep generative neural networks (DGNN), to design targeted regulatory DNA sequences, with the potential to revolutionize the field by enabling precise programmability of regulatory DNA.
Aim
To design and experimentally validate synthetic regulatory sequences for cell-specific gene expression control, using deep generative neural networks (DGNNs).
Methods
A comprehensive dataset for training the DGNNs will be developed by using a map of cell type-specific regulatory elements, such as enhancers, created by exploring single-cell chromatin accessibility data (ATAC-seq) and (RNA-seq). The regulatory sequences will be selected based on their specificity in controlling gene expression in therapeutically relevant cell types and validated through multiplexed single-cell enhancer reporter assays in stem cell-derived therapeutic cells.
Significance
If the project is successful, the AI-designed regulatory sequences might lead to greatly improved gene therapy by enabling precise control of gene expression in specific cell types.
Researchers
Nanna Holmgaard List (KTH), Talha Bin Masood (LiU)
Background
Sustaining almost all life on our planet and essential for visual perception, light plays a pivotal role in biology. At a molecular level, photo-responsive proteins are responsible for light-induced processes, whose biotechnological exploitations have already led to many life science breakthroughs. A deep understanding of these systems is crucial to understand photobiology and to engineer novel photoactive systems that can further advance life sciences.
Aim
The aim of the project is to combine automated quantum mechanics/molecular mechanics (QM/MM) workflows, topological data analysis, and visualization to investigate the workings of photoactive proteins. This three-pronged approach aims to decode complex data and understand not just “what happens” at the molecular level but also “why it happens.” The focus will be on proteins relevant to bioimaging and optogenetics.
Methods
The workflow for constructing reliable QM/MM models across sets of proteins will be optimized and improved significantly. The new models will also be used to create statistical and topological data analysis methods, capable of summarization, feature extraction, and robust comparison of time-evolving multifield data. An integrated visualization platform that combines analysis tools with innovative visual representations will also be developed.
Significance
The research will offer new insights into protein design factoring in photofunction with potential implications for a variety of technologies from biosensing to light-powered chemistry. Simultaneously, the project’s multifaceted analysis and visualization methods have broad applications, extending beyond biology to fields such as material and climate sciences.
WASP-DDLS Collaboration Projects 2021
During 2021, WASP and the SciLifeLab and Wallenberg National Program on Data-Driven Life Science (DDLS), the two largest research programs in Sweden, launched a joint call with the aim of solving ground-breaking research questions across their different scientific disciplines. In total, 15 applications were awarded grants for two-year projects.
Researchers
Joakim Andén (KTH), Erik Lindahl (SU)
Background
Proteins are essential biomolecules, acting in basically all cellular functions. Single-particle cryogenic electron microscopy (Cryo-EM) has traditionally been the main method for studying the morphology of large protein complexes. Advances in this technique in recent years are now allowing researchers to use cryo-EM to solve near-atomic-resolution macromolecular structures. However, available methods only work for rigid biomolecules and further knowledge about the inherent flexibility and dynamics of biomolecules are essential, not least in drug design.
Aim
To construct data-driven priors of atomic models trained using simulations obtained from molecular dynamics
Methods
Data driven priors in the form of deep neural networks (DNNs) will be used to construct atomic models. The priors will then be used to estimate the posterior distribution of atomic model trajectories given cryo-EM data.
Significance
The project will propose a framework to recover molecular motions and guide multiscale simulations of protein dynamics using cryo-EM data. This would serve as a catalyst for research both in machine learning and structural biology. An important application will be a better prediction of the effects of ligand binding to biomolecules, which is essential in drug design.
Researchers
Arne Elofsson (SU), Hossein Azizpour (KTH)
Background
All cellular functions are basically regulated by interacting proteins. The essential role of proteins in all living organisms are a result of their complex and variable three-dimensional structures, which in turn, enables their different functions. Increased knowledge about protein structures, functions and interactions are therefore fundamental in the understanding of basic cellular functions as well as alterations behind diseases.
In recent years, the AI/deep-learning system AlphaFold2 has radically improved the possibilities to retrieve highly reliable structures of single proteins only from its amino acid sequence. However, predicting protein binding and mechanisms of interaction remains a challenge.
Aim
To develop new deep learning tools to improve predictions of protein binding and interactions.
Methods
A new fold and dock protocol based on improved multiple sequence alignment and the AlphaFold2 tool has been developed, and will be developed further within this project. These tools, along with novel deep learning techniques, will be used to generate models of protein-protein interactions. In addition, the computational power in the AI/deep learning focused Berzelius SuperPOD will be used.
Significance
The tool developed in this project will be used to predict the structures of all known protein-protein interactions in open source databases, and thereby provide the biological community with a large library of protein interaction models. In the long term, this will increase the understanding of cellular functions as well as disease mechanisms.
Researchers
Mika Gustafsson (LiU), Rebecka Jörnsten (GU/CTH)
Background
Many common drugs work ineffectively for sub-groups of patients, as a result of the interplay between a multitude of small-effect genetic and epigenetic factors in complex diseases. New biotechnology methods, called omics, have made it possible to measure molecular imprints of a whole cell, which could be useful for development of more individualized therapies. Deep auto-encoders (DAEs), a type of artificial neural networks, are flexible non-linear dimension reduction methods, that recently have emerged as effective tools for summarizing high-dimensional complex genomics data.
Aim
To create a flexible multi-omic data integration tool that captures disease-specific structure across multiple levels of biological data to help identify processes related to disease outcome, severity, and response to treatment.
Methods
DAEs will be developed with latent spaces constrained by biological side information such as cellular pathways, that can be combined into Deep translational networks (DTNs). The DTNs are further constrained with biological information on the samples, e.g., disease subtypes or clinical outcome.
Significance
More advanced data-driven data-integrative methods will be essential in biology as multi-omics single-cell data sets are rapidly emerging. This project will illustrate how flexible integration of multiple data sources can lead to new insights into disease processes, which is the key to individualized treatment strategies.
Researchers
Joakim Jaldén (KTH), Wei Ouyang (KTH)
Background
Cellular stress responses are shown important in normal human physiology as well as in disease mechanisms. Live cell fluorescence microscopy allows visualization of morphological changes, revealing cellular states. Convolutional Neural Networks (CNN) enable protein pattern recognition and cell segmentation of microscopic images. However, successful training of deep learning models requires capturing not only the major population of cells, but also the minority of rare cells.
Aim
To perform early identification and active tracking of cells with rare cell fates including apoptosis, senescence, and drug resistance, by using reinforcement learning in the live cell imaging setting.
Methods
Two computational elements will be developed:
1) A method that is sensitive enough to identify cell fate groups based on their morphological changes and stress response pattern.
2) A strategy for a self-driving microscope to reduce phototoxicity and maximize the chance to acquire data points for less represented rare cell populations.
Significance
The self-driving system will enable rapid on-the-fly cell fate prediction, automatic microscopy control, and active tracking and acquisition for rare cell populations. The work will provide a framework for modeling the entire cell in a purely data-driven manner. Such digital cell models will further allow in-silico cell experiments which can directly contribute to accelerated drug discovery for a wide range of diseases.
Researchers
Sven Nelander (UU), Rebecka Jörnsten (GU/CTH)
Background
Each year, approximately 1300 new cases of brain tumors are diagnosed in Sweden. A better understanding of the mechanisms behind cellular growth of different tumors are essential to develop new, targeted therapies against the diseases. New biotechnological methods such as single cell sequencing and gene editing show promise as tools to explore brain tumor biology. The data generated by these methods are complex and require new analytical methods.
Aim
To develop a new method to further uncover key genes and cellular pathways behind brain tumor growth.
Methods
CRISPR sequencing will be used for large-scale analysis screening of brain tumor xenografts. Thereafter, AI-models based on neural networks, that integrate and structure the CRISPR-Seq results in relation to other databases will be utilized to reveal therapeutic targets and mechanisms.
Significance
The project will address several challenges including the formulation of a new class of AI models, based on structured and adaptive regularization. The project can increase our understanding of invasive brain tumor growth and the role of AI methods for genomic data interpretation. This will improve our capacity to identify in vivo relevant disease genes, thereby enabling development of new therapies specifically targeting certain tumor diseases.
Researchers
Martin Rosvall (UmU), Beatrice Melin (UmU)
Background
The biobanks and the extensive medical registers in Sweden comprise a goldmine for precision medicine research. However, exploiting the benefits of these resources is limited by the strict regulation of sensitive personal data (GDPR) and the complexity of growing amounts of data.
Aims
Making biobank data accessible by developing new solutions to overcome the obstacles of handling sensitive personal information and analyzing complex data.
Methods
The new biobank infrastructure PREDICT built from the Västerbotten Intervention project will provide data and implement the results coming out from our planned analyses. We will develop a machine-learning approach for data de-identification and evaluation of the de-identified biomedical data based on risk for re-identification and data quality, allowing data sharing and recycling unrestricted by GDPR.
In addition, we will develop methods for fast, interactive biobank data visualization and dimensionality reduction for longitudinal data from repeated samples donated by the same individual over time, enabling a broad research community to access the data.
Significance
Making the biobank data accessible will propel precision medicine research, advance its clinical use, and ultimately improve population health and survival in major endemic diseases.
Researchers
Kevin Smith (KTH), Theodoros Foukakis (KI)
Background
Breast cancer is the most common cancer for women and around 1,500 patients die each year in Sweden due to the disease. Regular mammographic screening has been demonstrated to decrease mortality by 20% to 40%, however, this method has limited sensitivity for some tumor types. ScreenTrust, an AI-based approach, has been developed for estimating breast cancer risk from screening images with some success. However, all models have been based on Convolutional Neural Networks (CNNs) that only accept a single image as input data.
Aim
To develop a new class of neural networks for breast cancer risk assessment that considers information from other views and historic images, to improve early detection and select patients for more sensitive screening methods.
Methods
Vision transformers (ViTs) will be used, which can outperform CNNs on standard vision tasks. ViTs provide a powerful way to combine multiple mammographic views from a patient. In addition, the ViT can treat an individual image or an entire patient’s clinical history as a sentence and learn which words (or regions) to pay attention to for the task at hand.
Significance
Reliable AI-based methods to estimate breast cancer risk offer a potential solution, which could allow hospitals to offer effective personalized screening regimens and care to women, thereby increasing breast cancer survival.
Researchers
Petter Brodin (KI), Dimos Dimarogonas (KTH)
Background
Immune systems in humans vary a lot between individuals. At the same time, the composition of cells in the blood is stable within an individual over time in the absence of perturbations such as an infection. To understand higher order functions in this complex system and its regulatory mechanisms, simultaneous analyses of all cell populations are required. Technological advances now allow such analyses.
Aim
To develop a network model for the immune system responses.
Methods
A novel single-cell genomics approach established in the Brodin lab will be used where all immune cell populations are stimulated and analyzed in whole blood cultures. Cell composition will be modulated with individual cell types depleted and the consequent functional responses by remaining cells analyzed. Using state-space techniques, a network model of different immune system responses will be modelled. This can be used to design strategies on how to improve immunomodulatory therapies, by utilizing and expanding leader-selection and pinning control methodologies.
Significance
Understanding cell-cell dependencies and the regulatory network of immune cells will allow human immune responses to become more predictable. Precise immunomodulatory treatments may be devised using data-driven precision interventions, targeting the most important nodes in the immune cell network of a given patient.
Researchers
Tino Ebbers (LiU), Ingrid Hotz (LiU)
Background
Personalized medicine is at the center of all discussions of future medicine. The promise is to enable earlier diagnoses, better risk assessments, and optimal treatments by providing patient-specific care and treatment. The foundation for this is the generation of realistic patient models, which are largely based on high-quality imaging techniques.
Consequently, medical imaging data is continuously growing in size and complexity, where every generation has resulted in more data per exam. However, it also gives rise to many challenges in terms of handling and accessibility of a huge amount of complex imaging data.
Aim
To provide a pipeline for the next generation of personalized heart models.
Methods
Data will be collected by using the image acquisition technology of the future and methods that support an efficient and effective analysis of the data for diagnosis and model building, integrated in a visual data browser with high-quality rendering, will be developed.
Significance
Expertise from two Postdocs, one with a medical engineering background and the other, with Data analysis and visualization experiences, will be combined in this project. Jointly they will generate detailed heart models, extract flow and muscle strain tensor data harvesting the PCD-CT data. From the generated data, a database according to FAIR principles will be built. The visual data browser will support interactive exploration of the database with high quality rendering of anatomy, blood flow and heart muscle strains, and advanced search function.
Researchers
Mats Karlsson (UU), Bo Bernhardsson (LU)
Background
In the context of precision medicine, the purpose of pharmacometric modeling is to make predictions on an individual basis, based on known possible covariates. Traditionally, covariates have been restricted to demographic parameters (age, weight, etc.), but lifestyle (smoking, exercise, etc.) and omics data (SNPs, etc.) are vastly expanding the possible parameter space, presenting both challenge and opportunity.
Ultimately predictive performance is limited by the amount of available training data, how informative the training data is, and how much of inter-patient variability that can be explained by the covariates present in the data set. One key challenge lies in understanding or learning causality dependences and using them to establish a sound covariate model.
Aim
To develop methods to simultaneously learn the structure and parameters of covariate models and to improve pharmacometric predictors suited for individualized precision medical therapies.
Methods
Two machine-learning methods, Knowledge-based regularization (artificial neural network (ANN) and kernel models) and Learning Low-dimensional mechanistic and casual relations, needs to be developed. Datasets from Propofol, the Diabetes Registry, and the Multiple Sclerosis Registry will be considered for this project.
Significance
Pharmacometric predictions constitute a cornerstone in precision medicine. The existence of well-established national quality registries puts us in an internationally unique position to lead the development of data-driven pharmacometrics modeling, with the prospect of strengthening Sweden’s position within research on the area, and to inspire further collaboration in-line with the objectives of the KAW DDLS initiative.
Researchers
Tuuli Lappalainen (KTH), Bo Wahlberg (KTH)
Background
Understanding causal regulatory networks of the cell is one of the most fundamental gaps in our current biological knowledge. While we know that genomic information is transmitted to cellular functions via complex regulatory networks, we have limited knowledge of how genes regulate each other.
Previous approaches to tackle this question have been unsatisfactory: gene co-expression studies and equivalent correlation-based approaches lack directionality of causation, genetic data is sparse, and classical molecular biology of specific pathways lacks the necessary scale.
Aim
To develop novel computational methods to infer causal regulatory networks in human cells from existing and upcoming CRISPR gene knockdown data sets.
Methods
Modern causal learning models will be adapted to the high-dimensional perturbation data from single-cell genomics experiments to model gene regulatory interactions. The methods will be extended to handle perturbations of thousands of genes, and include uncertainty estimates to distinguish between no causal effect or insufficient data. Single-cell gene perturbation experiments with CRISPR will be used to validate inferred causal networks.
Significance
The combination of novel statistical methods and modern functional genomics toolkit – with scalable CRISPR perturbations coupled with single-cell molecular readouts – provides exciting novel opportunities to infer causal regulatory networks of the cell. Application of these methods to diverse data sets will allow us to characterize basic biology of cellular function, empower design of maximally informative experiments, and discover specific regulatory pathways underlying genetic risk for complex disease.
Researchers
Fredrik Lindsten (LiU), Sebastian Westenhoff (UU)
Background
Determination of biomolecular structures has led to scientific breakthroughs and innovations in molecular biology and new treatments for diseases. The recently developed deep-learning-based system AlphaFold 2 has improved the possibilities for structure prediction of single proteins. However, several challenges remain, including prediction of conformational heterogeneity.
Machine learning models are commonly trained from large datasets, but once trained they are unable to adapt to constraints or auxiliary, instance-specific data during inference. This is particularly problematic when reliable uncertainty quantification regarding their predictions is required.
Aim
To develop novel algorithms to include instance-specific experimental constraints in machine learning models, to bridge the gap between AI predictions and experimental observations, thereby providing new tools for producing reliable, hybrid “predicted/experimental” protein structural ensembles.
Methods
Initially, AI-generated structure predictions for complex of phytochrome proteins will be scored against low-resolution cryo-electron-microscopy (EM) data. Hybrid structures will be obtained, which are expected to significantly increase the resolution compared to the pure data. The solution obtained with experimental constraints will be re-fed into the predictive model. In a second step, probabilistic modeling, and uncertainty quantification (UQ) will be performed to predict conformational heterogeneity.
Significance
The novel hybrid predicted/experimental protein structures will combine the best of cryo EM (high reliability, single particle technique, measures conformational heterogeneity) and predicted protein structures (high resolution, readily available). The novel AI methods are widely applicable, examples include materials design, drug discovery, and personalized medicine.
Researchers
Alexander Schliep (GU/Chalmers), Pär Matsson (Sahlgrenska Academy)
Background
Machine learning (ML) and Artificial Intelligence (AI) have been a remarkable success in improving small molecule drug discovery, from generating novel molecular structures to suggesting synthesis pathways. While small molecules interacting with proteins are the most frequently used drug modality today, alternatives are increasingly explored to address unmet clinical needs. Particularly, oligonucleotide therapeutics – i.e., drugs based on chemically modified short RNA/DNA sequences – are opening new opportunities in disease areas where traditional drugs have failed.
Aim
To predict thermodynamic effects of novel chemical modifications to oligonucleotides, predict the impact of chemical conjugation on the thermodynamics of therapeutic oligonucleotide binding, and enable federated, privacy-preserving learning of thermodynamics prediction.
Methods
Driven by the sample data for thermodynamics of DNA-DNA hybridization, ML models which can serve as the basis for transfer to chemically modified oligonucleotides, will be developed.
Available data for specific chemical modifications will provide input for transfer learning. Extensions to novel modifications, and to combinations of different modification types, will be additionally based on in silico simulations where experimental data is sparse. By using existing data, one of the goals of the project is to predict the impact of conjugation and select suitable conjugates dependent on oligonucleotide sequence and targeted cell type. Finally, competing entities will be allowed to learn ML models for tasks such as off-target binding prediction from pooled data of oligonucleotide binding without compromising privacy of drug candidate data.
Significance
The proposal will greatly expand the speed of exploration of novel individual and combination strategies of chemical modification and conjugations in the design of novel oligonucleotide drugs. The proposal will also suggest a way for pharmaceutical companies and other parties to pool data without sacrificing privacy.
Researchers
Mathias Uhlén (KTH), Andreas Kerren (LiU)
Background
There is a need for a functional genome-wide annotation of the protein-coding genes to get a deeper understanding of mammalian biology. Present genome-wide annotation tools are useful, but require arbitrary cut-offs, commonly obtained via black-box computational models. This may hinder the ability of the analyst to make an informed decision regarding what are relevant fold-changes and detection limits for the underlying transcriptomics data.
Aim
To develop a new data-driven strategy for exploring whole-body co-expression patterns, using interpretable machine learning with the help of interactive visualization techniques, that support informed decisions, leading to better predictions and improved trustworthiness of the results.
Methods
The new data-driven strategy will be based on interpretable unsupervised learning of whole-body co-expression patterns, supported by state-of-the-art visual analytics. Interactive guiding of the clustering process will be used to explore the gene expression landscape in humans and other mammalian species, to create a whole-body map of all protein-coding genes in all major cell types, tissues and organs.
Significance
The new interactive clustering strategy will improve the quality of the classification of all protein-coding genes according to their expression “landscape”, allowing distinct clustering of genes related to tissues and/or functions, such as testis or muscle contraction. Since the data will be publicly available and visualized in the Human Protein Atlas, it will be of world-wide use for the research community.
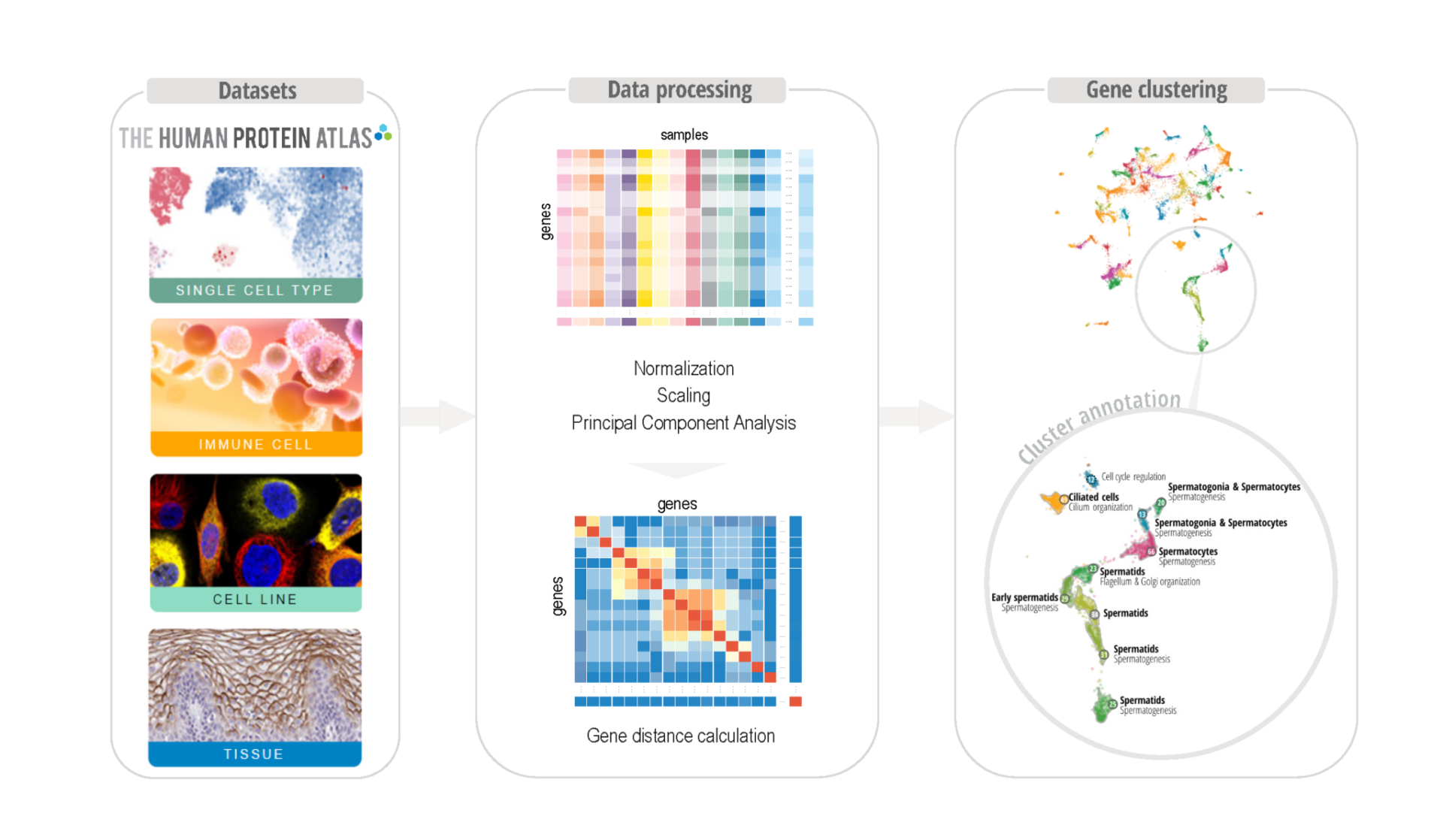
Clustering pipeline used to develop an unsupervised gene clustering framework supported by visual analytics. The data will be publicly made available and visualized in the [Human Protein Atlas].
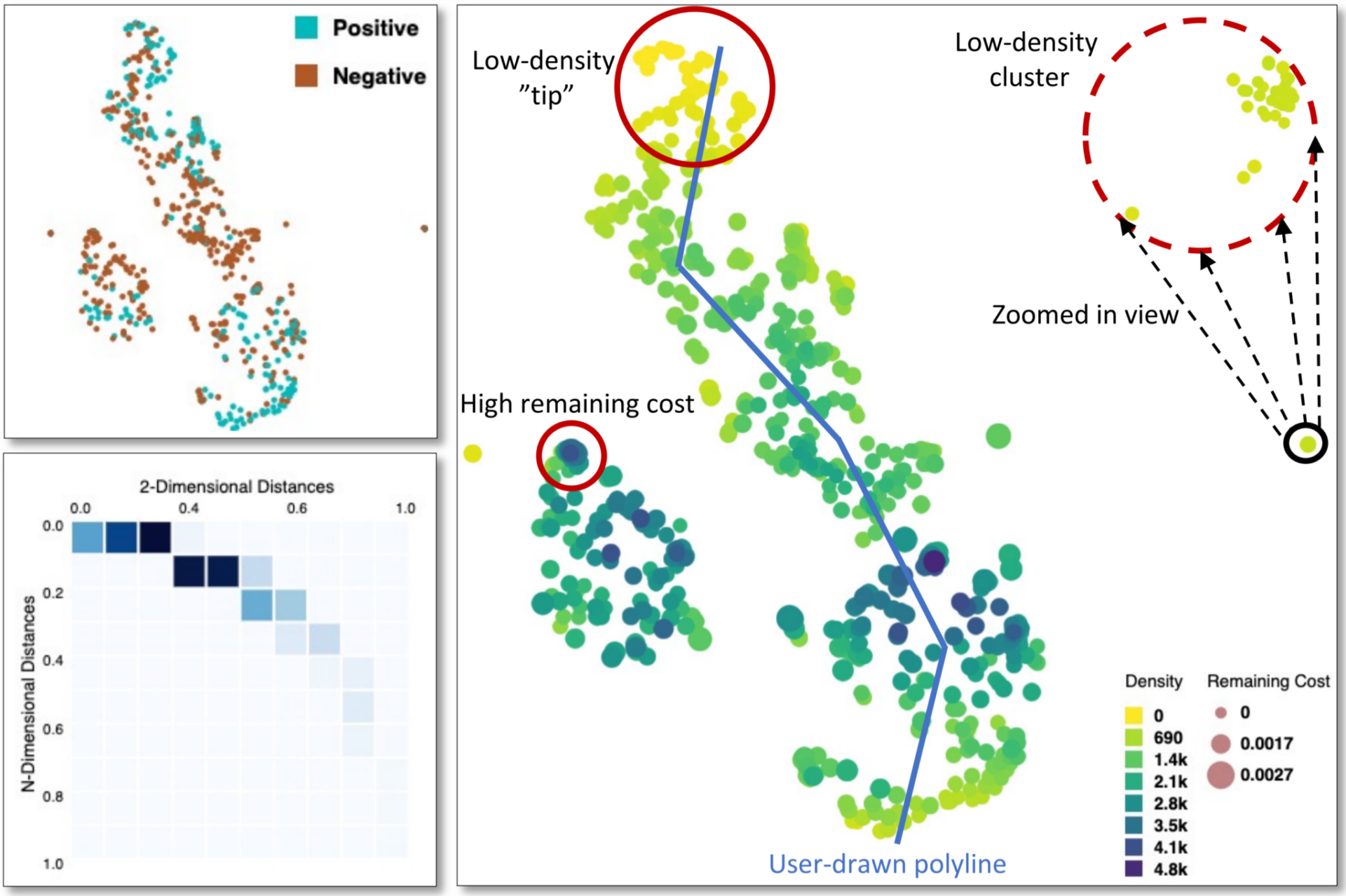
Example of a visual analytics tool, called [t-viSNE], for the visual exploration of t-SNE projections that enables analysts to inspect different aspects of their accuracy and meaning. Similar approaches will be developed in the project to provide interpretable and interactive clustering, fine-grained quality analysis of the clustering, as well as better cluster annotation by using visual analytics.
Researchers
Björn Wallner (LiU), Alexey Amunts (SU)
Background
Protein-protein interactions underlie the dynamic processes of all living cells. Until very recently the main tool to directly visualize those interactions has been cryo-EM. However, this technique is limited to the most stable complexes.
At the moment, the protein structure field are experiencing a revolution with the development of powerful computational structure prediction tools, AlphaFold, which is powered by advanced deep learning neural networks, that can compete with the experimentally obtained crystal structures.
Remarkably, AlphaFold works well for protein complexes even when it is trained on individual proteins. The reason for this is somewhat unclear but protein-protein interactions are not that different from protein interactions within a single chain. However, folding large protein complexes, with multiple linkers, involving transient interactions will still be a challenge.
Aim
To develop new tools to investigate large dynamic multi-component systems.
Methods
AlphaFold will be used to systematically predict protein-protein interactions along the ribosomal assembly pathway, by analyzing previously unpublished high-resolution structures of assembly intermediates of human ribosome assembly in mitochondria. This is done to optimize the AlphaFold pipeline and to provide a proof of principle.
Using previous results, transient interactions and structural dynamics will be explored by retraining the Evoformer and the Structural Module. Finally, a system that is optimized to use raw experimental data or electron densities, in addition to the evolutionary and structural information, will be developed.
Significance
Due to highly flexible nature of many other cellular assembly systems, and the fundamental importance of the biological problem proposed here, the methods pioneered in this project are expected to be visible and therefore applied also beyond the ribosome assembly problem and become a widely used tool for multi-protein macromolecules.
NTU–WASP Collaboration Projects
One of our main international partners is Nanyang Technical University (NTU), Singapore. Together with some of their top researchers and doctoral students we have formed NTU-WASP Collaboration Projects.
Objective
Improve the security of existing machine learning algorithms against real-world attackers
Targeted Problem
Data poisoning attacks on optimization-based supervised learning algorithms and their defense.
Approach
- Understand vulnerabilities of supervised learning algorithms. We will find blind spots of a broad family of supervised learning algorithms.
- Design optimal defense strategies against data poisoning attacks. We will develop two defense frameworks: The detection framework aims to accurately and timely detect the occurrence of poisoning attacks and the mitigation framework aims to effectively reduce the influence of attacks on victim learning models.
- Improve solution robustness and evaluate proposed methods. We will refine the detection and mitigation frameworks and propose new algorithms to compute optimal defense. We will design evaluation frameworks and validate solutions on real-world data sets
PIs
Bo An (NTU), Chew Lock Yue (NTU), Christos Dimitrakakis (Chalmers), Devdatt Dubhashi (Chalmers)
Objective
To develop co-evolutionary algorithms for reinforcement learning in multi-agent systems
Approach
- Demonstrate the techniques on adaptive scheduling of an intelligent autonomous bus network at NTU campus
Preliminary Results
- Proof of concept for elevator scheduling (Figure 1)
- Data analytics on campus bus network (Figure 2)
- Preliminary results in simulations (Figure 3)
PIs
Chew Lock-Yue (NTU), Bo An (NTU), Mikael Johasson (KTH)
Objective
Interactive training of deep networks for vision-based autonomous systems. Reproducible machine learning of navigation and path following in autonomous vehicles such as cars and drones
Targeted Problem
Generate learning data in an interactive, dynamic, and adaptive way.
Approach
- Virtual paths, i.e., synthetic environments are projected onto the ground.
- Small-scale vehicles drive autonomously in these synthetic scenarios and acquire their training data in a dynamic environment that can adapt to the learning progress.
- Allowing for full control of all environmental parameters, for appropriate
- Generation of training data by photo realistic rendering of virtual images during real navigation and path following
PIs
Michael Felsberg (LiU), Kai-Kuang Ma (NTU)
Objective
Computing capacity in edge locations and the wireless access are managed separately. However, delivering the full potential of MECs requires that edge locations and wireless networks be managed in concert
Targeted Problem
To design new resource allocation methods to improve and unify the management of Mobile Edge Clouds (MECs).
Approach
- Game theory will be used to analyze the optimal strategy of each player, thus verifying that the goals of the devised mechanisms are met.
- A player interaction protocol will devised and validated using simulations.
- Development of mathematical models and algorithms to efficiently solve the network and computing resource allocation for MECs.
PIs
Erik Elmroth (UmU), Dusit Niyato (NTU)
Objective
Improved algorithms for sensor fusion, localization and coordination for search and rescue operations and surveillance applications
Targeted Problem
Centralized and distributed sensor fusion for target localization, reliable relative localization and collaborative control and task planning of the agents
Approach
- Environmental modelling of stationary and moving targets.
- Centralized and distributed fusion estimation methods for multiple robots.
- Reliable relative localization in GPS denied environments.
- Distributed optimization and hybrid control from local temporal logic specifications for multi-robot systems
PIs
Fredrik Gustafsson (LiU), Dimos Dimarogonas (KTH), Hu Guoqiang (NTU)
Objective
Develop advanced techniques and tools for visualizing different aspects of machine learning jointly in the same framework.
Targeted Problem
Visualization of the distributions of the input data with effective filters, development of new data structures for handling high dimensional and heterogeneous data and networks, and investigation of how to present and analyse the results with respect to the input data.
Approach
- Our approach is to supply a holistic view of a machine learning system and provide interactive visualization that facilitates human involvement and various stages of machine learning.
- We start with deep learning and computer vision tasks as the initial application domain in the area of facial reconstruction.
- We combine new and traditional data visualization methods and will develop a project demonstrator.
PIs
Anders Ynnerman (LiU), Jianmin Zheng (NTU)
WASP Expedition Projects
A concept with high gain/high risk, targeted expeditions with a specific challenging goal – that is what the WASP Expedition Projects are about. Rather than developing long term thematic frameworks these two-year projects focuses on taking leaps in a specific area. The projects run across departments and universities and consists of two PIs and up to four Postdocs. The following projects are active.
PIs: Emil Björnson, LIU, Pontus Giselsson, LU
PIs: David Broman, KTH, Magnus O. Myreen, CTH
PIs: Iolanda Leite, KTH, Jana Tumova, KTH
PIs: Benoit Baudry, KTH, Erik Elmroth, UMU
PIs: Bo Bernhardsson, LU, Maria Sandsten, LU
PIs: Giuseppe Durisi, CTH, Katerina Mitrokotsa, CTH
PIs: Anders Hansson, LIU, Bo Wahlberg, KTH