Intelligent Cloud Robotics for Real-Time Manipulation at Scale
To endow robotic systems with capabilities to reliably and safely grasp and manipulate the vast variety of objects encountered in both industrial and everyday home settings, significant interdisciplinary breakthroughs are required before such systems can be deployed. While machine learning relying on large scale image, text and audio data is progressing at rapid pace, robotic manipulation research has in comparison been starved for data since data acquisition on a single robotic system is expensive and slow. This project tackles challenges in this domain by focusing in particular on the emerging paradigm of Cloud Robotics, where robots can communicate over a network to share observations and jointly learn from past observations.
Long term impact
We focus on addressing fundamental scientific challenges in the domain of cloud robotics for robotic manipulation. The project establishes a Swedish network of excellence in the emerging field of Cloud Robotics for robotic manipulation by combining interdisciplinary expertise in cloud computing, control and robotic manipulation between KTH, Lund and Umeå University and under participation of network partners such as the WARA Robotics, WARA Ops, ABB and Algoryx AB. As part of its mission, the NEST is also training the next generation of subject experts in this field in Sweden. As part of this effort, a testbed system of multiple robotic arms hosted at KTH will in particular be established to allow for experimentation at scale.
Scientific Presentation
This project focuses on five key challenges in cloud robotics related to overcoming the scaling bottleneck of robotic manipulation and to developing robust data-driven robotic manipulation methodologies that benefit from the cloud robotics paradigm and network effects of scale:
- Goal 1: scaling and operation of a first large scale cloud robotic manipulation demonstrator system with more than 100 robotic arms based on the open source CloudGripper design developed at KTH, to enable the evaluation of methodologies and training data generation at scale.
- Goal 2: The development of fault-tolerant methodologies, rigorous experiment design and methodologies for testing and real-time edge-cloud control for such systems at scale.
- Goal 3: The development of methodologies for reasoning about asymptotic scaling behavior, robustness and transfer-learning of robotic manipulation policies trained at large scale.
- Goal 4: The development of federated and continual machine learning approaches suitable for robotic manipulation in an edge-cloud setting.
- Goal 5: The development of distributed edge-cloud resource allocation methodologies based on joint utility addressing the particular challenges of cloud robotics applications
Background
Autonomous robotic systems that can reliably grasp and manipulate objects in their environment in order to perform complex tasks “in the wild” have been the goal of robotic manipulation research for decades, promising breakthrough productivity and safety improvements to industrialized and aging societies with potential applications ranging from industrial automation to robotic health- and elderly care and personal robotic assistants. While approximately 2.7 million industrial robotic systems are estimated to be in operation worldwide as of 2020, these systems currently do not yet benefit from a network effect at scale as they are mostly manually programmed and do not share information or learn collaboratively in a large scale data-driven manner. As a result, the predominant industrial robotic manipulation applications are still very limited to specific tasks in controlled environments such as industrial welding, pick-and place and palletizing tasks.
Recent progress in machine learning applications, particularly in fields such as large language models, speech recognition and computer vision, have been powered by a confluence of factors chiefly related to developing systems at scale. In particular, performance breakthroughs have been enabled by scalable machine learning algorithms, large scale training data collection and processing and cloud computation that enables dynamic resource allocation depending on the machine learning task requirements.
In this interdisciplinary project spanning machine learning, robotics, cloud-computing and real-time control, we focus on achieving a breakthrough in the foundational algorithms, machine learning and system design requirements for scaling networked, distributed, robotic manipulation systems to a large network of cloud-connected robots. We envision this network to continuously collect very large scale manipulation training data and to dynamically learn from past experience using federated machine learning.
Our approach aims to allow robots to balance between centralized machine learning in the cloud and local processing of information using the computational resources of each individual robot while incorporating real-time control and network bandwidth constraints.
Methods
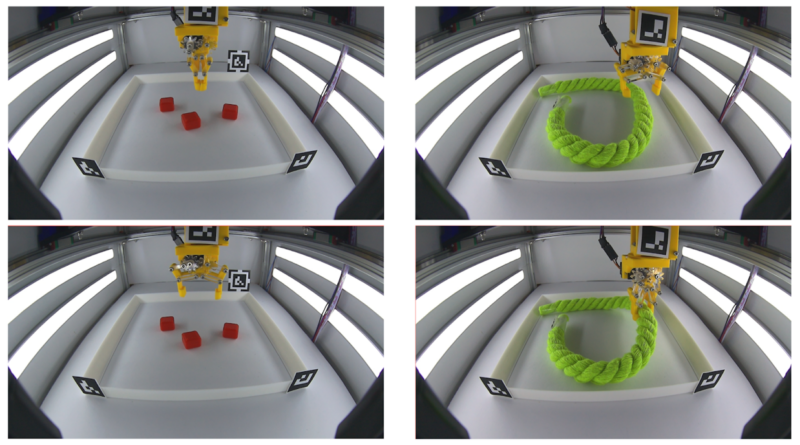
Figure 1: Open Source CloudGripper robots developed at KTH which will form an experimental test-bed for cloud robotics methods and software developed by the NEST and which we intend to scale to more than 100 robots during the lifetime of the project.
The project brings together an interdisciplinary team from KTH Royal Institute of Technology, Umeå University and Lund University with expertise in Machine Learning, Control, Cloud Computing and Robotic manipulation to tackle the above research challenges. A diverse set of methodologies ranging from distributed and federated machine learning to novel robust control approaches and experiment design, resource allocation methods and transfer learning will be investigated by the team to tackle the project’s scientific goals. The NEST furthermore has a strong focus on real world evaluation and testing of algorithms in the context of a cloud-robotics test-bed which we will scale to an installation of more than 100 robot arms as part of the project.
Environment & Community Building
The NEST’s supporting partners include WARA Robotics, WARA Ops, ABB, Algoryx AB. We furthermore intend to build a strong international network of researchers and industry interested in cloud-robotics and hope to open our cloud-robotics test-bed also to external collaborators when the system has reached sufficient maturity.